How to Build an AI Agent: A Step-by-Step Builder Guide
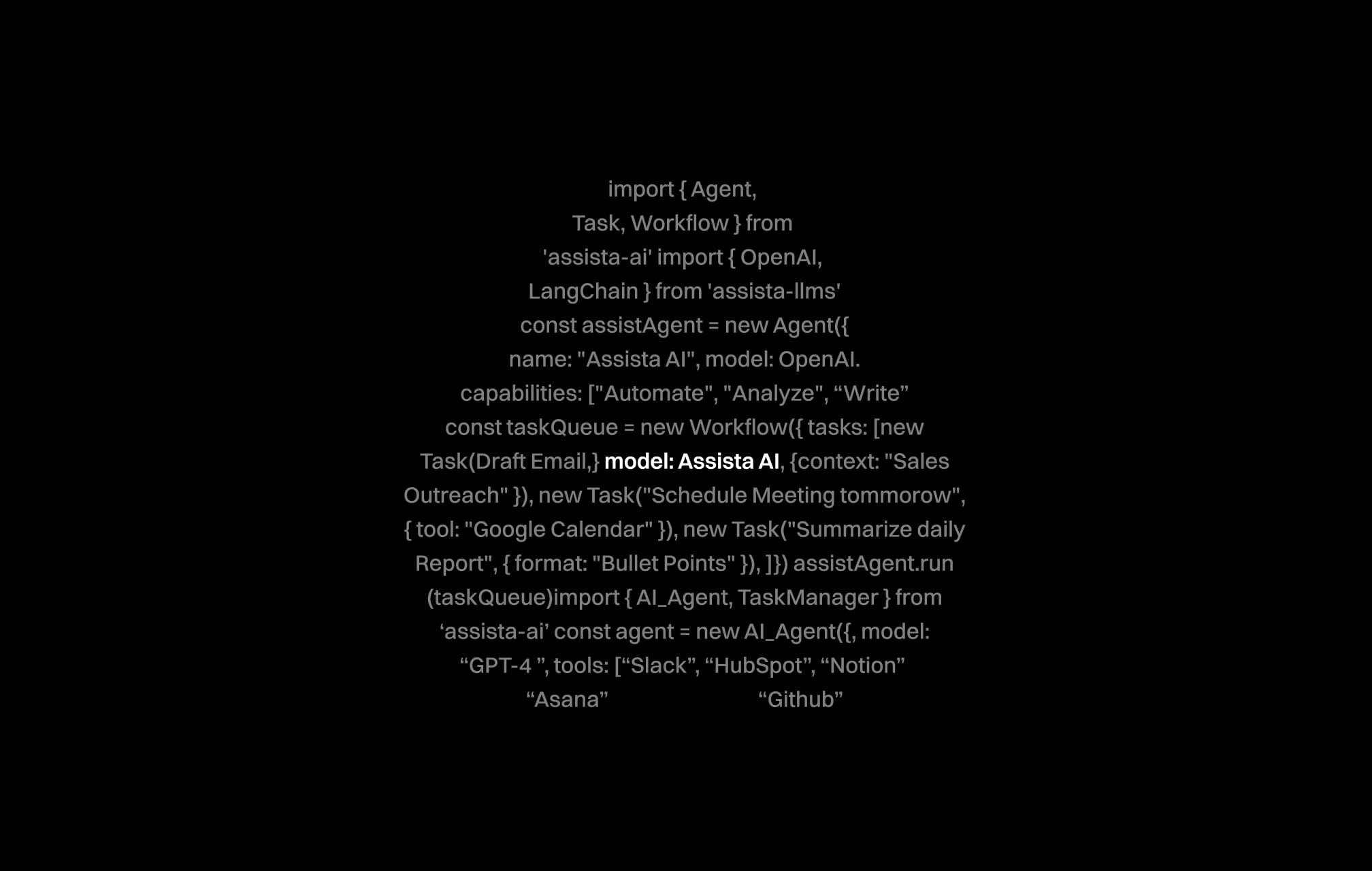
Welcome to Your AI Agent Journey
If you’ve ever wondered how to create an AI assistant that can handle routine tasks or enhance your team’s productivity without needing a technical degree, you are in the right place. In this guide, we explore the AI agent builder process that transforms simple ideas into sophisticated automated systems. Whether you're a digital worker or a small business owner, knowing how to build an AI agent is invaluable. Here, you will learn strategies and step-by-step instructions that address common challenges, helping you navigate the world of AI agent construction from planning to deployment. We highlight modern development tools and practical examples so you can see how tasks like email drafting, lead retrieval, and report generation are automated by an AI. With clear instructions and actionable tips, this article ensures you leave with a strong foundation in building your own AI assistant. The techniques outlined capitalize on proven best practices, and we even offer real-world integration examples with platforms like Gmail, Trello, and Google Sheets. Get ready for an insightful journey into the realm of AI agent building.
Grasping the Fundamentals of Building an AI Agent
This section lays the groundwork for understanding what it means to build an AI agent. To start with, you need to know what an AI agent is and why it is a game changer for teams managing multiple digital workflows. An AI agent is essentially a software entity programmed to perform specific tasks by processing natural language inputs, executing automated actions, and interacting with various platforms.
Why Build an AI Agent?
Building an AI agent offers numerous benefits. It enables non-technical users to interact with complex software through a simple chat interface. This helps in:
- Reducing repetitive manual tasks
- Enhancing workflow efficiency
- Integrating multiple tools such as Gmail, HubSpot, and Google Sheets without the need for coding
Studies show that automation can improve operational efficiency by up to 30%. For instance, a recent report on productivity improvements by Harvard Business Review highlights the tangible benefits businesses experience with automated workflow solutions.
Key Concepts to Know
Before you embark on your AI agent builder journey, it’s important to understand the following concepts:
- User Intent: Determining what your user needs the AI agent to do.
- Natural Language Processing (NLP): The ability of your agent to understand and process human language.
- Integration and Workflow Automation: Linking the AI agent with productivity tools such as Gmail and Trello to automate multiple tasks.
Grasping these fundamentals sets the stage for a successful build process and highlights how valuable an AI agent builder can be in modern digital workspaces. Next, we explore how to effectively plan and design your AI agent.
Planning and Designing Your AI Agent
Effective planning and design are the foundation of a successful AI agent. This stage helps you clarify your goals, outline core functionalities, and create a roadmap that guides the build process. By carefully planning your project, you ensure that every step of your agent is purposeful and addresses a genuine productivity gap in your workflow.
Defining Objectives and Use Cases
Start by identifying the key tasks your AI agent should perform. Typical use cases include:
- Email management and drafting responses in Gmail
- Lead retrieval from HubSpot
- Task updates and scheduling in Asana
- Generating reports from Google Sheets data
Establishing clear objectives helps you focus on features that will have the greatest impact on your work processes. It is important to note that without a well-defined scope, your project might become too complex or lose its effectiveness.
Mapping Out Workflow Integrations
Integration planning is crucial; you may want your AI agent to interact directly with productivity platforms like Google Sheets, Slack, and HubSpot. These integrations allow your agent to perform multi-step tasks seamlessly. Consider the following integrations:
- Link your agent to Google Sheets for data analysis and report generation.
- Integrate with Slack for team communications and automated updates.
- Utilize HubSpot to manage lead generation and customer support workflows.
For an extensive list of supported tools, visit the integrations page. With these planning details, you can now move on to the construction phase, where the actual building takes place.
Step-by-Step AI Agent Builder Process
The building phase is where ideas turn into reality. This section takes you through a detailed, step-by-step process of constructing your AI agent. With a clear outline, even non-technical users will find the process manageable.
Step 1: Select Your Development Environment and Tools
Choosing the right development environment sets the tone for a streamlined build process. Many professionals favor modern software solutions that offer supportive libraries and plugins. While coding is not a necessity for leveraging the AI agent builder, knowledge of fundamental concepts can help. Consider using platforms that offer one-click OAuth integration, similar to those found in productivity tools like Google Calendar and LinkedIn.
Step 2: Designing the Workflow Architecture
This phase involves several key decisions:
- Define the interaction model: Will your agent use a natural language processing module to interpret commands?
- Determine integration points: Identify which apps and services will be connected.
- Plan the decision-making logic: This is where you establish how your agent will process information and execute tasks.
Having a clear architecture plan ensures every component of your AI agent interacts effectively with others. You can reference articles on the blog for additional insights on workflow design and automation strategies.
Step 3: Building the Core Functionalities
Begin by creating modules that perform simple tasks and gradually combine them into more complex workflows. Consider the following building blocks:
- Data retrieval and processing from platforms like GitHub for project updates.
- Natural language processing for interpreting user queries.
- Response generation systems that draft emails or complete forms.
This iterative process allows you to test small parts of your system individually. For example, if you’re focusing on task management integrations, testing with tools such as Asana can provide useful feedback before full integration.
Step 4: Testing and Refinement
Once the core functionalities are built, rigorous testing is the next logical step. This involves both automated testing scripts and real-world trials with typical use cases. Testing helps identify any glitches or potential improvements. Consider establishing a testing checklist:
- Check all integrations for correct data exchange
- Run user interface tests to ensure natural language text is interpreted as intended
- Validate the accuracy of data processing from external tools
Feedback from beta users is invaluable. For an external perspective, a recent study by Forbes highlighted that systems undergoing rigorous user testing show a 25% higher success rate upon launch.
Step 5: Launching Your AI Agent
After testing and refining your system, it’s time to deploy. At this stage, ensure all integrations are fully functional and the workflow operates as expected. Provide clear documentation and instructions for end users. You might even consider a soft launch to a small group before a full organizational rollout.
As you prepare for launch, remember to focus on collecting user feedback so you can continuously improve your AI agent. If you’re ready to start building, check out the sign up page for a user-friendly platform that empowers digital workers with AI capabilities.
Evaluating, Testing, and Deploying Your AI Agent
This stage covers one of the most critical aspects of your project – evaluating the performance of your AI agent. You have built the core functionalities, now it’s essential to monitor and refine the system through user feedback and metrics.
Monitoring Performance and User Engagement
After deployment, use data analytics to monitor how your AI agent interacts with users. Focus on these key performance indicators:
- User satisfaction ratings
- Task completion rates
- Speed and accuracy of data retrieval
Implement periodic user surveys and feedback sessions. Insights from these evaluations can guide you to optimize your agent further. Additionally, revisit the integration points on platforms such as Google Calendar and LinkedIn to ensure that everything remains current and fully functional with API updates.
Iterative Testing and Refinement
Remember that no AI agent is perfect out of the gate. Consider these strategies for continuous improvement:
- Schedule regular testing intervals (weekly, monthly) to catch potential issues early.
- Utilize user analytics to pinpoint specific pain points in the workflow.
- Implement A/B testing for newly added features, comparing performance against your current baseline.
The iterative testing process helps you perfect the agent over time. Check out changelog for updates and methodologies that further optimize the performance of your agent.
Deployment Best Practices
When your AI agent passes all tests, deploy it gradually to a larger user base. Follow these best practices:
- Roll out the agent in phases to monitor system performance at each stage.
- Offer clear user guides and support documentation.
- Establish a feedback channel for immediate troubleshooting and suggestions.
Successful deployment is not the final step—it’s the beginning of a continuous cycle of evaluation, refinement, and upgrade. This iterative approach ensures that your AI agent remains effective and relevant over time.
Applications, Future Trends, and Tips for Enhancing Your AI Agent
With your AI agent now built and deployed, it’s time to explore its various applications and future enhancement strategies. This section focuses on leveraging your creation to its fullest, highlighting real-world use cases and trends in automation.
Real-World Use Cases
AI agents today serve multiple industries, from marketing to customer support. Some practical applications include:
- Sales Automation: Retrieve leads from HubSpot, update Salesforce records, and generate performance reports without manual intervention.
- Customer Service: Automate common queries and route tickets directly from platforms like Zendesk to your support team.
- Project Management: Sync tasks across Asana, Trello, and Monday to maintain consistent data flow and effective communication.
For instance, companies have used AI agents to create weekly performance dashboards by connecting Google Sheets and Slack. The benefits include reduced administrative workload and improved decision-making speed. You may explore more case studies on the blog to see success stories that inspire innovative approaches.
Future Trends and Innovations
Looking forward, the evolution of the AI agent builder process continues to be dynamic. Trends shaping the future include:
- The integration of advanced natural language understanding capabilities.
- Greater collaboration between multiple AI agents to handle complex tasks.
- Enhanced security and compliance features as AI becomes pivotal in managing sensitive information.
Research by reputable organizations emphasizes that businesses leveraging cutting-edge AI integrations can experience significant increases in productivity and efficiency. Staying informed on these trends through sources like Forbes can help you keep your AI agent ahead of the curve.
Enhancing Your AI Agent Over Time
Once launched, continuous improvement is key to maintaining relevance and performance. Consider these enhancement strategies:
- Regularly update your integration modules to match API changes from connected platforms.
- Incorporate machine learning feedback loops to refine the agent’s decision-making process.
- Expand functionalities gradually, such as adding new integrations available on the marketplace.
Additionally, keep an eye on user feedback and performance metrics to prioritize feature upgrades. The journey from a basic AI agent to a fully optimized digital assistant is gradual but immensely rewarding when timed correctly with evolving business needs and technology improvements.
Your Next Steps and Final Thoughts
Now that you have learned the step-by-step process for building an AI agent, it’s time to put theory into practice. The path from planning to deployment is filled with opportunities to innovate and expand the capabilities of your digital assistant. Focus on constant evaluation and adopt a roadmap that embraces continuous improvement.
To recap, this guide has covered:
- The fundamental principles of AI agent development.
- Effective planning techniques and workflow integration strategies.
- A practical, step-by-step process with testing and deployment best practices.
- Real-world applications and future trends to fuel enhancements.
By mastering these insights, you can confidently approach the challenges of digital workflow automation. Whether you are using your AI agent for lead generation, project management, or customer service, the benefits are clear: reduced repetitive tasks, increased productivity, and a smarter approach to managing your daily operations.
If you are ready to build an AI agent that revolutionizes your workflow, remember that the process is iterative and rewarding. Make sure to revisit our internal resources such as the about page and explore additional tips on the blog for ongoing inspiration. Your journey into building a powerful, AI-driven assistant is just beginning, and the tools you need are closer than ever.
Embrace the challenge, stay curious, and transform the way you work—one intelligent step at a time.
We invite you to explore further innovations and join the community of users pushing the boundaries of what’s possible with an AI agent builder. Your next step could be the spark that launches a new era of productivity in your organization.
Join over 1,400 professionals who reclaimed their time and sanity by letting AI handle the busywork.